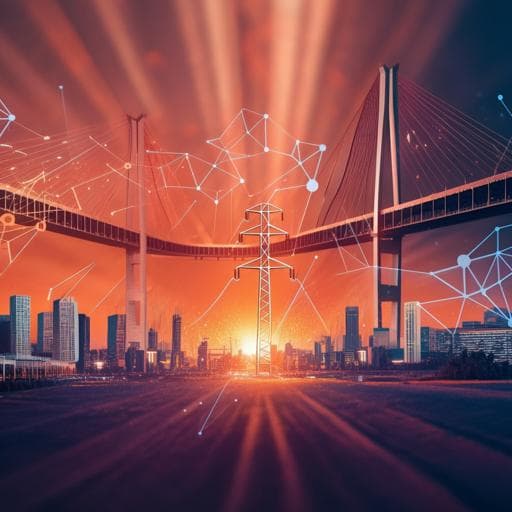
Engineering and Technology
Development of a civil infrastructure resilience assessment framework and its application to a nuclear power plant
T. K. Singhal, O. Kwon, et al.
Explore the groundbreaking Civil Infrastructure Resilience Assessment Framework (CIRAF) developed by Tarun K. Singhal, Oh-Sung Kwon, Evan C. Bentz, and Constantin Christopoulos from the University of Toronto. This innovative framework uses a Bayesian Network approach to assess the seismic fragility and resilience of civil infrastructure systems, evaluating factors such as functionality loss and recovery time. Delve into its application through a case study of a nuclear power generation system and uncover effective upgrade strategies.
Playback language: English
More Research from Authors
Discover additional research by the author.